Istanbul: More equitable access to the taxi system
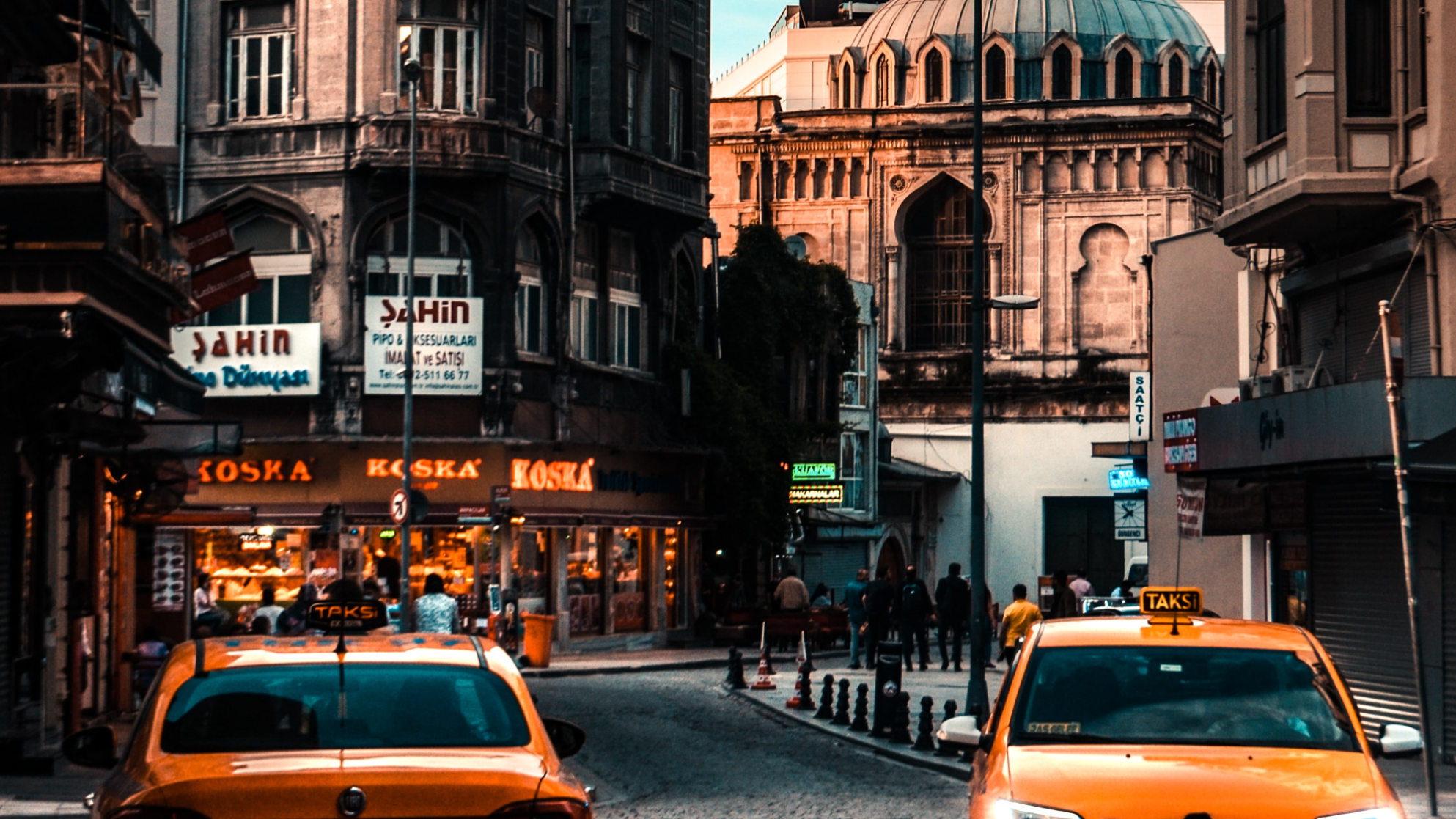
The Challenge
In Istanbul, 1.7 million of the 32 million daily journeys are made by taxis. While the urban population has grown, the number of taxis (around 20k) remained stable since the 90s. Hence, the city faces a deficit in taxis while their integration in last mile transportation gains importance in order to reduce the need for private car ownership.
At the same time, compliancy issues reduce the service quality of the Istanbul taxi fleet. Taxi drivers tend to select passengers based on their own judgment and turn down many passengers (53.8%) as they prefer longer distance over short distances trips (6.9%) defined as trips up to 4.5km. Moreover, often tourists are prioritised over local residents due to the possibility to claim a higher price. A common trick pulled on non-local passengers is to make trips longer than necessary to charge a higher fee. As well, manipulation of the taximeter is a common practice.
All this leads to restricted accessibility to the taxi system, insecurity for the transport participants and ultimately to an inequitable mobility offering. To mitigate compliance and safety issues, taxis, as well as minibuses and buses are requested by law to have in-cabin cameras installed. A large amount of video material including time stamps and location data are available to the municipal transport authority. However, an automated solution to analyse the data in a privacy-compliant manner and to process complaints efficiently was lacking.
The Solution
A privacy-compliant AI-application was developed by DataJiro to analyse the data and eventually to detect fraudulent behaviour. While the authorities had access to the video material, geo-location data and time stamps, still no reliable information was available about the actual length of the trips.
Analysing the video material the exact time and location when passengers enter and leave the taxi vehicle defining the start and end of the trip, could be identified. Having this data available, the actual trip length as well as the most efficient route could be automatically calculated. Comparing the actual trip length with the most efficient route, fraudulent behaviour could be detected.
Moreover, a score based on the ratio of short vs. long-distance trips was consequently revealed for each of the analysed taxis. Taxis with a higher ratio of long distance trips were identified as potential incompliancy cases.
In addition to identifying fraudulent behaviour, the application can generate various reports on each vehicle's travel duration, passenger count, routes, efficiency, and compliance with traffic rules.
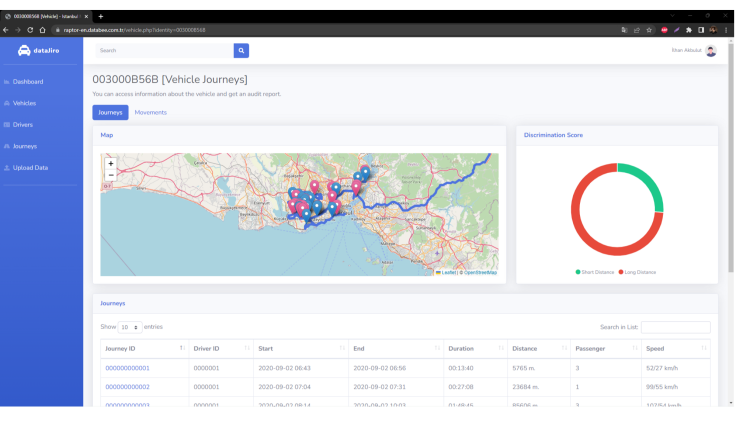
Making an impact
- 2,000 hours of journeys from 40 randomly selected vehicles, collected over 139 days have been analysed.
- Journey/route data analysis with exceptionally high accuracy (99.7%)
- It was determined that approximately 12% of these trips involved fraudulant behaviour against passengers
The detected incidents were formatted for reporting to relevant authorities who will conduct investigations and determine the appropriate course of action.
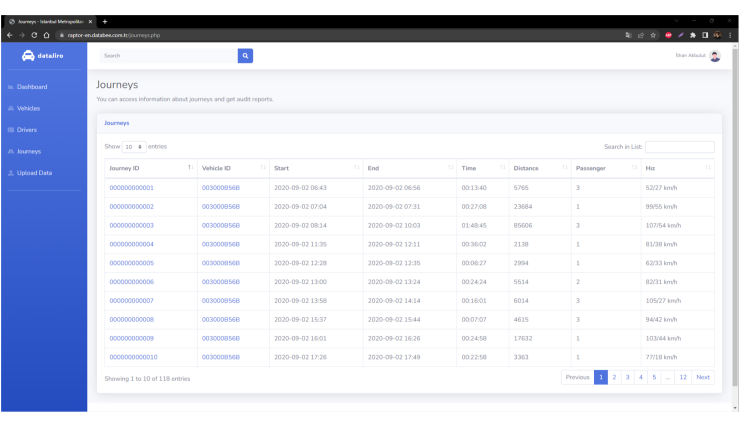
Lessons learnt
- The potential of AI applications for the common good.
- The importance of continuous monitoring and proactive efforts to enhance and ensure fairer mobility offerings for everyone.