Identifying individual mobility patterns in Munich
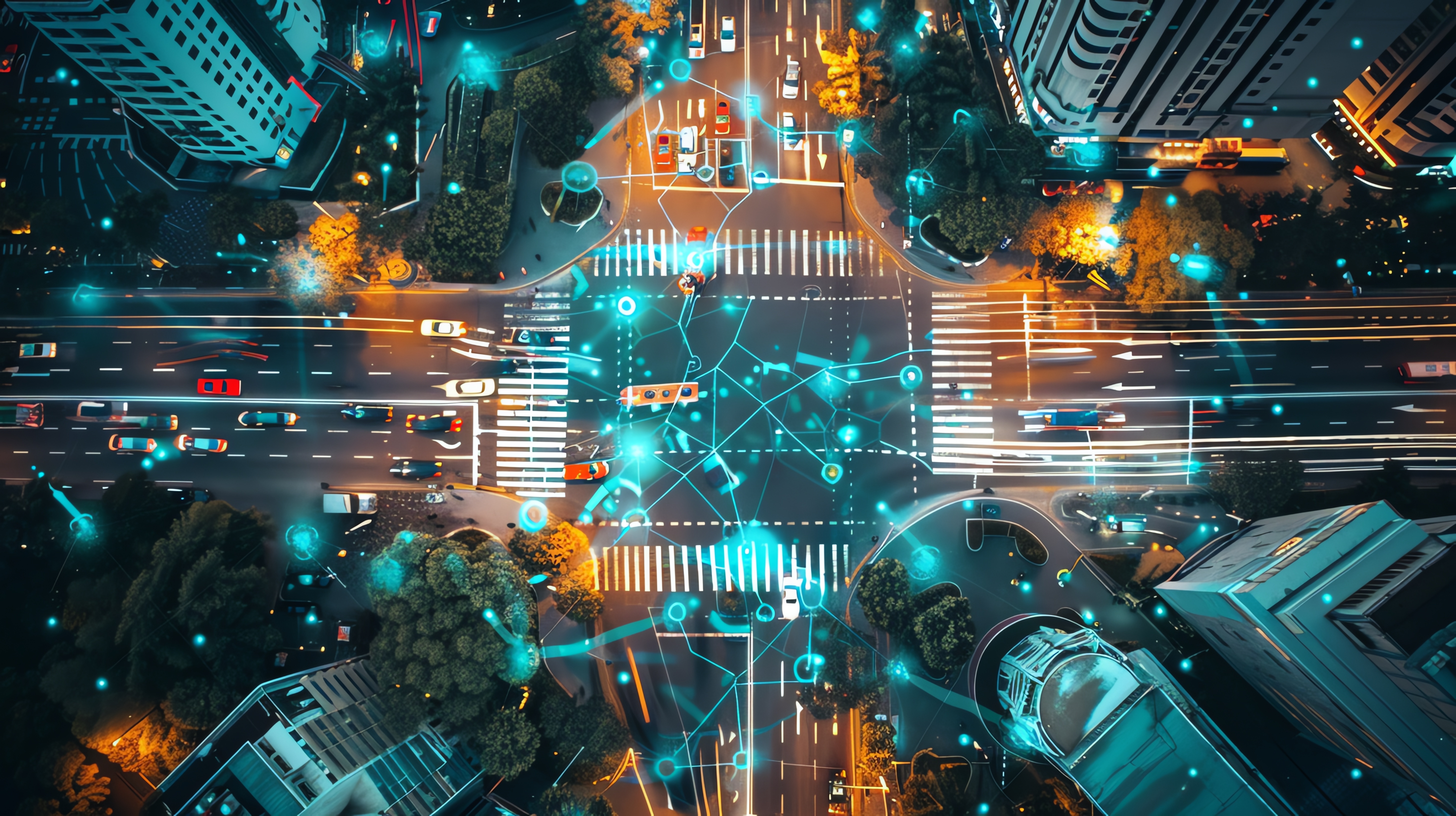
The Challenge
The city of Munich, with its population of 1.5 million, faces significant environmental and traffic challenges due to the increasing number of inhabitants and private vehicles. This rise has resulted in higher CO2 emissions and congestion.
Additionally, around 400,000 commuters travel to Munich daily from surrounding areas, exacerbating the situation. To enhance the public transport services provided by Stadtwerke München (SWM) and Münchner Verkehrsgesellschaft (MVG), public transport operator in Munich, it's essential to understand how passengers use various modes of transport. This knowledge is crucial for attracting more people to environmentally friendly transportation options.
Currently, data on public transport usage is limited. Passenger counts are collected through counting devices installed on a subset of public transport vehicles, covering only 15-60% of the fleet. This data allows for calculating long-term averages but cannot provide specific information for particular days or times. Cell-phone data providers real-time movement streams but cannot track short distances (under 1 km) or differentiate between modes of transport. App-based solutions suffer from insufficient and non-representative user bases, making accurate projections difficult.
The Solution
To address these challenges, Transcality has created a digital twin of the multi-modal transportation system that can support operations and planning of public transport in Munich.
The solution from the ETH Zurich spin-off, Transcality, integrated data from various sources, including the automatic passenger counts from Münchner Verkehrsgesellschaft (MVG) vehicles, daily public transport fleet schedules, traffic measurements from inductive loop detectors and openly available map data to ensure high data quality. Furthermore, this integration combined historical passenger count data with the static macroscopic model used by MVG.
Transcality’s solution allowed the automated creation of a detailed and fully calibrated multimodal mesoscopic simulation model, the digital twin, based on the integrated data. This model provided a more granular view of public transport usage such as passenger-specific origin-destination tables and ensures seamless integration into MVG’s existing data flow.
The digital twin allows for scenario management and traffic flow forecasting. It enables to visualise historical, current, and forecasted traffic conditions, providing deep insights into travel behaviour.
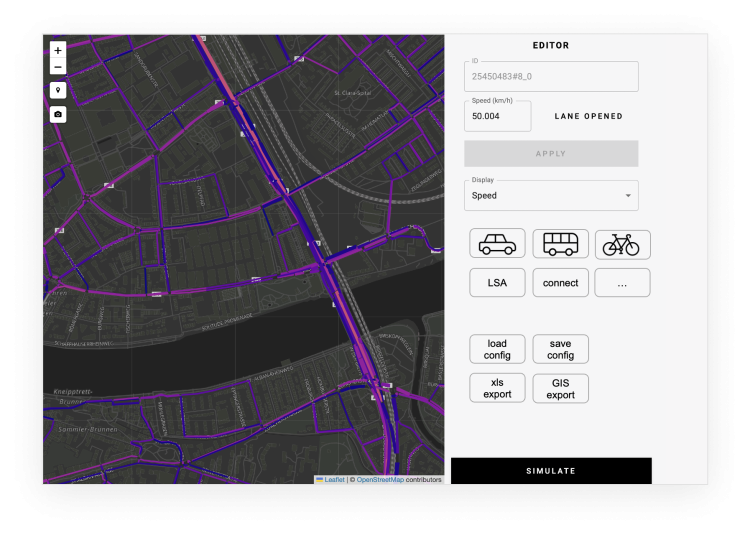
Making an impact
The deployment of the digital twin for Munich has yielded significant results:
- Enhanced understanding of travel behaviour: The model provided detailed insights into how many people travel, at what times of day, and via which modes of transportation. This information is invaluable for optimising public transport services and encouraging the use of environmentally friendly options.
- Improved planning and operations: By forecasting traffic flows and visualising different scenarios, the digital twin aids in better planning and operational decision-making. This leads to more efficient use of resources and improved service for passengers.
- Data-driven decision making: The integration of various data sources and the development of a robust mesoscopic model enable SWM and the City of Munich to make informed decisions based on accurate and comprehensive data.
- The passenger counts were automated from MVG including bus, tram and metro in Munich
- Weather data was monitored on an hourly basis
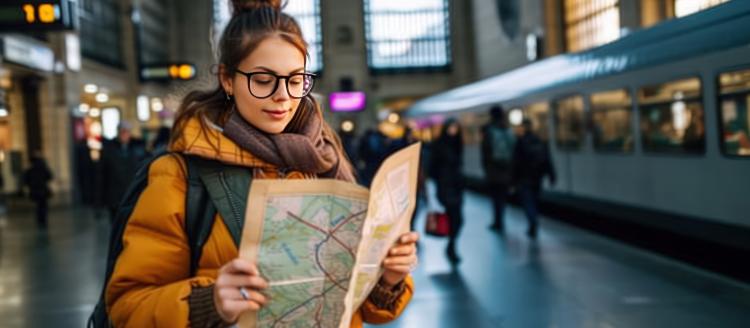
Lessons learnt
For the success of this project, several circumstances were key:
- Sufficient historical data on passenger counts across the entire public transport fleet over a long period of time was provided by MVG.
- Development of a concept that meets the requirements of the city. Thanks to the great communication between the involved parties, meeting the requirements was successful.
- Development of a concept that can be transferred to other cities. This was achieved by relying on openly available data and tools.